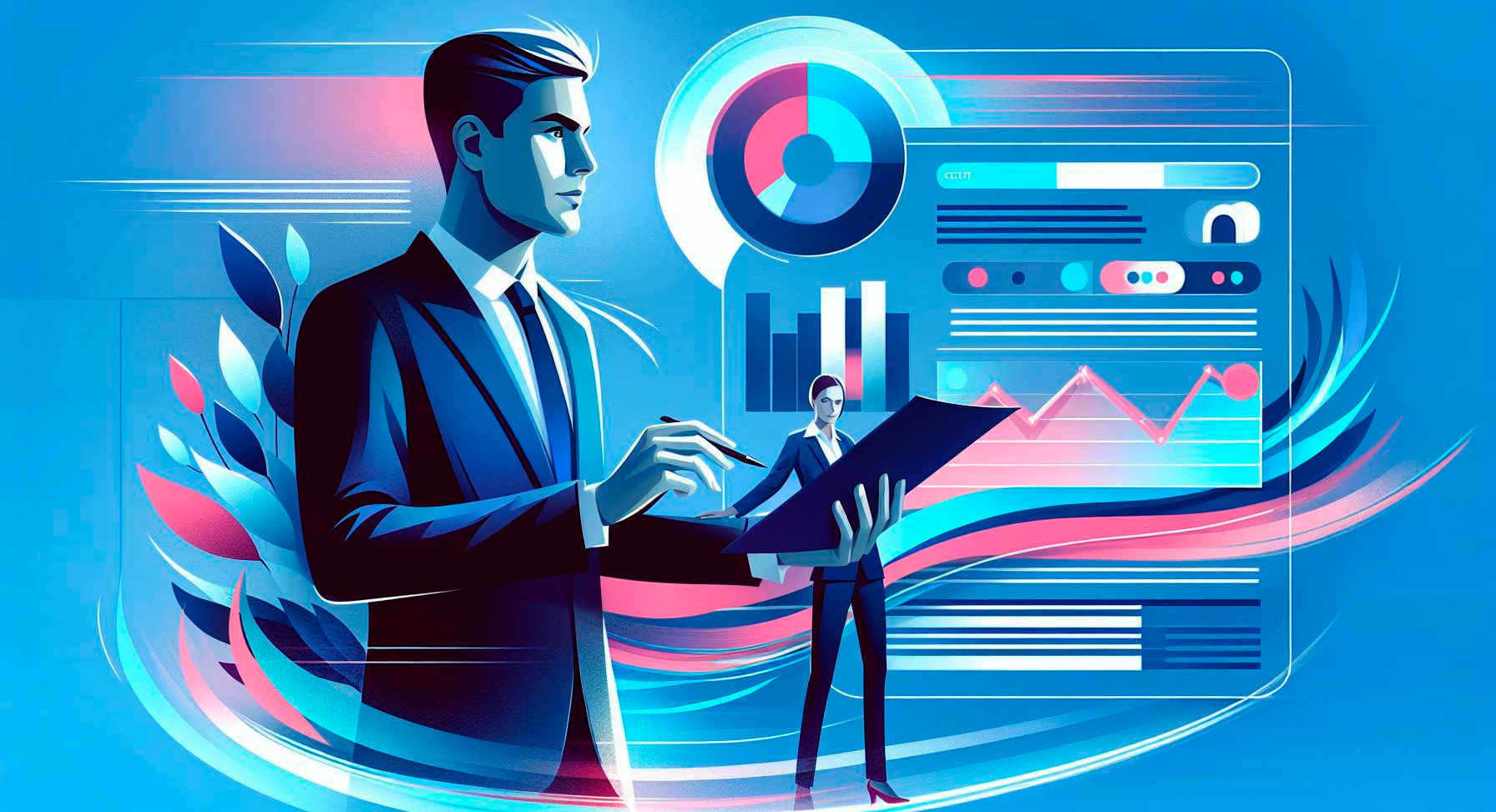
Optimizing Credit Risk Model Validation: Best Practices and Insights
Validating credit risk models isn’t just about ticking boxes; it fundamentally impacts financial institutions’ decision-making. With accurate credit risk assessments, banks can confidently extend credit and set terms aligned with borrower reliability. This not only safeguards the institution’s interests but enables better financial decisions.
Regulatory compliance is a must. Ensure your credit risk models meet key requirements by regularly conducting back-testing, benchmarking, and stress testing. These methodologies help identify discrepancies, improve accuracy, and ensure your models withstand various economic scenarios. Always maintain data integrity and continuously monitor risks and limitations for robust model validation.
Emerging trends like machine learning and AI bring new challenges and opportunities to the validation process. While they offer improved predictive accuracy, they complicate model interpretability and necessitate high-quality data. Balance these advancements with effective validation frameworks to enhance credibility and maintain regulatory compliance. By staying ahead of these trends, your institution can navigate future complexities with confidence.
How Does Credit Risk Model Validation Impact Financial Institutions’ Decision-Making?
Credit risk model validation impacts financial institutions’ decision-making by ensuring the models predicting loan defaults are accurate and reliable. You need this validation process to have confidence in your models, ensuring they function as intended.
By validating your credit risk models, you can:
- Make Informed Lending Decisions: Accurate assessments of borrowers’ creditworthiness help you decide on extending credit and setting terms.
- Manage Financial Risk: Identifying model weaknesses allows you to adjust strategies to mitigate risks and avoid losses.
- Comply with Regulations: Meeting regulatory requirements prevents penalties and maintains good standing with regulatory bodies.
- Improve Model Performance: Ongoing validation reveals inaccuracies, helping you refine models for better predictions and more reliable decision-making.
In the end, validating your credit risk models ensures they provide dependable insights, supporting better financial decisions and safeguarding your institution’s interests.
What Are The Key Regulatory Requirements For Credit Risk Model Validation?
You need to ensure your credit risk models meet these key regulatory requirements:
First, you should verify the conceptual soundness of your model by checking its theoretical foundations and the statistical and mathematical methods used.
Next, regularly conduct validation activities. You should back-test against historical data, benchmark against external models or industry standards, and perform stress tests to observe how the model performs under extreme conditions.
Ensure internal validation by conducting ongoing monitoring to verify compliance with internal models, especially those used for calculating minimum capital requirements. This includes assessing the probability of default (PD), loss given default (LGD), and exposure at default (EAD).
You should ensure the accuracy and completeness of the data used in your model to maintain data integrity. It is also crucial that you validate the parameters within the model for accuracy and relevance in parameter estimation.
Establish strong governance policies and an independent validation process, particularly for models impacting financial statements like CECL. This forms part of good governance and control.
You must provide annual validation reports to regulatory bodies, detailing your model’s accuracy and reliability, ensuring transparency in your reporting.
Continuously monitor and address any risks stemming from model limitations or new techniques, such as machine learning, under model risk management. Also, you should comply with guidelines set by bodies like the ECB, FED, and OCC, maintaining stable and accurate model predictions over time.
As a final point, you need to ensure your credit risk models are conceptually sound, regularly validated, and comply with regulatory standards while maintaining transparency and strong governance.
What Methodologies Are Effective For Testing And Validating Credit Risk Models?
Effective methodologies for testing and validating credit risk models include back-testing, benchmarking, stress testing, and incorporating machine learning techniques.
You should consider back-testing, where you evaluate your model’s predictions against historical data. This helps you identify discrepancies and improve model accuracy. Benchmarking is also essential; you compare your model’s performance against other external models or industry standards to ensure it meets or exceeds established benchmarks.
Stress testing subjects your model to extreme, yet plausible, economic scenarios. This ensures your model is robust and reliable under adverse conditions. You can also utilize advanced machine learning algorithms like logistic regression, random forest, and support vector machines to handle large datasets and improve predictive accuracy.
To gain better insights into borrowers’ creditworthiness, incorporating alternative data sources like social media sentiment, satellite imagery, and transactional data can be incredibly beneficial. Cross-validation, where you split your data into multiple parts to train and test your model multiple times, ensures consistency and reliability in your predictions.
Using explainable and ethical AI enhances model transparency and addresses biases, thereby improving overall trust and effectiveness in your credit risk assessments.
Bringing it all together – by employing these methodologies, you can ensure your credit risk models are accurate, reliable, and capable of supporting effective risk management strategies.
Why Is Data Quality Important In Credit Risk Model Validation?
Data quality is crucial in credit risk model validation because it directly impacts the accuracy and reliability of the models used to predict credit risk. High-quality data ensures you can accurately estimate the probability of default, loss given default, and exposure at default, all of which are essential for effective risk management. Here’s why:
- Accurate Predictions: Good data quality leads to reliable model outputs, helping you accurately assess the risk of borrowers defaulting on loans.
- Risk Management: Reliable data allows you to set appropriate risk limits, allocate capital efficiently, and take timely corrective actions to mitigate risk.
- Regulatory Compliance: High-quality data ensures your models comply with regulatory standards, avoiding penalties and maintaining your institution’s reputation.
- Fraud Prevention: Clean data helps you detect and prevent fraudulent activities by ensuring the data is free of errors, biases, and inconsistencies.
- Operational Efficiency: With accurate data, you can streamline your credit risk management processes and improve decision-making.
All things considered, by prioritizing data quality in your credit risk model validation, you enhance the overall stability and performance of your credit risk management strategies, supporting accuracy, compliance, and operational efficiency.
What Are The Primary Challenges Faced During Credit Risk Model Validation?
One major challenge you face when validating credit risk models is the lack of sufficient data. Without enough relevant data, it’s hard to see if your model works accurately. Another challenge is dealing with the assumptions and limitations of the models themselves. If you don’t understand these well, your validation results might be incorrect.
The complexity of the models is another big issue. They often use many variables and techniques, which can be tough to fully understand and validate. Data quality and integrity are crucial too. If your data is inaccurate or incomplete, the validation process can lead to misleading conclusions.
Regulatory compliance adds another layer of difficulty. You need to ensure your models meet various legal and regulatory standards, which can be quite demanding.
Finally, you also have to handle data imbalances, where there might be too few samples to represent certain credit risk scenarios. This can make it tough to ensure your model predictions are fair and accurate.
Lastly, you face many hurdles, from inadequate data and complex models to regulatory demands and data imbalances, when validating credit risk models.
You may also like
Archives
Calendar
M | T | W | T | F | S | S |
---|---|---|---|---|---|---|
1 | 2 | 3 | 4 | 5 | 6 | |
7 | 8 | 9 | 10 | 11 | 12 | 13 |
14 | 15 | 16 | 17 | 18 | 19 | 20 |
21 | 22 | 23 | 24 | 25 | 26 | 27 |
28 | 29 | 30 | 31 |
Leave a Reply